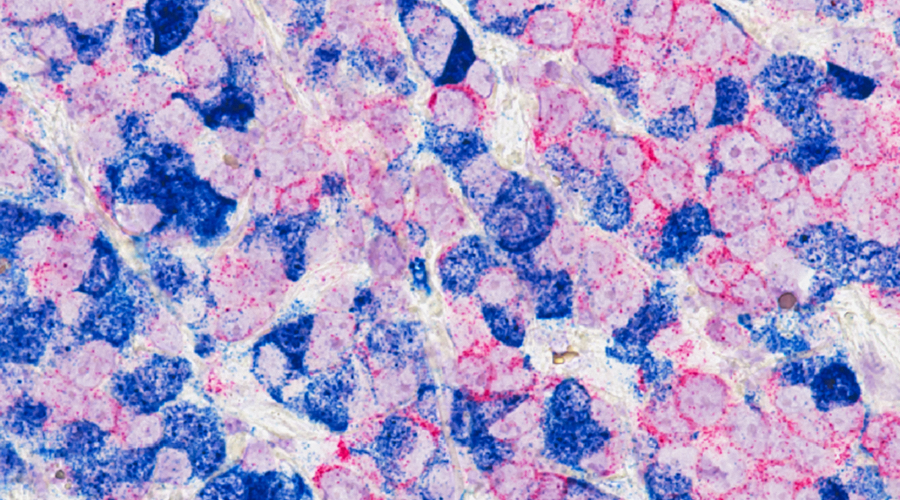
Breast Cancer: Novel 3D Imaging and Mammograms
Developing a Novel 3D Imaging Tool for Intraoperative Specimen Assessment during Breast Cancer Resection: A Pilot Clinical Evaluation Study
PI: Xiaoqin (Jennifer) Wang, MD; Co-I’s: Richard Gibbs, MD; Han Qiong, MD; Yu Zhang, PhD; Nathan Jacobs, PhD; and Jinze Liu, PhD.
Xiaoqin (Jennifer) Wang, MD, Assistant Professor in Radiology with a subspeciality in breast imaging, is principal investigator of this retrospective 3D imaging breast cancer resection research. The study is funded by an American Cancer Society grant. Presently mammogram is the standard screening tool for the general population and has been proven to reduce mortality in clinical trials. Mammography, however, is not perfect, diagnosis can be missed, especially in patients with dense breast. Dr. Wang proposes to develop a deep learning tool, using imaging which will help radiologists detect breast cancer more accurately, improving radiologist’s efficiency, improvement in clinical operations, but more importantly improved patient care benefiting more women. Application of this new tool, known as artificial intelligence (AI) in breast cancer screening will transform the screening challenges, moving clinical practice toward reduced call back rates. Lower call backs can eliminate unnecessary medical costs for redundant biopsies, decrease patient’s psychological stress, and ultimately may decrease the risk of developing invasive breast cancer in millions of women. Women who are needlessly called back yearly leading to neglected mammography screening. Research is ongoing; to date she and her research team have reviewed over 2,000 patient cases, to which interim findings are published in the following abstract: “Classification of Whole Mammogram and Tomosynthesis Images Using Deep Convolutional Neural Networks”, IEEE Trans Nanobioscience 2018 Jul,17(3):237-242.doi: 10.1109/TNB 22018.2845103. Epub 2018 June 7, https://www.ncbi.nlm.nih.gov/pubmed/29994219
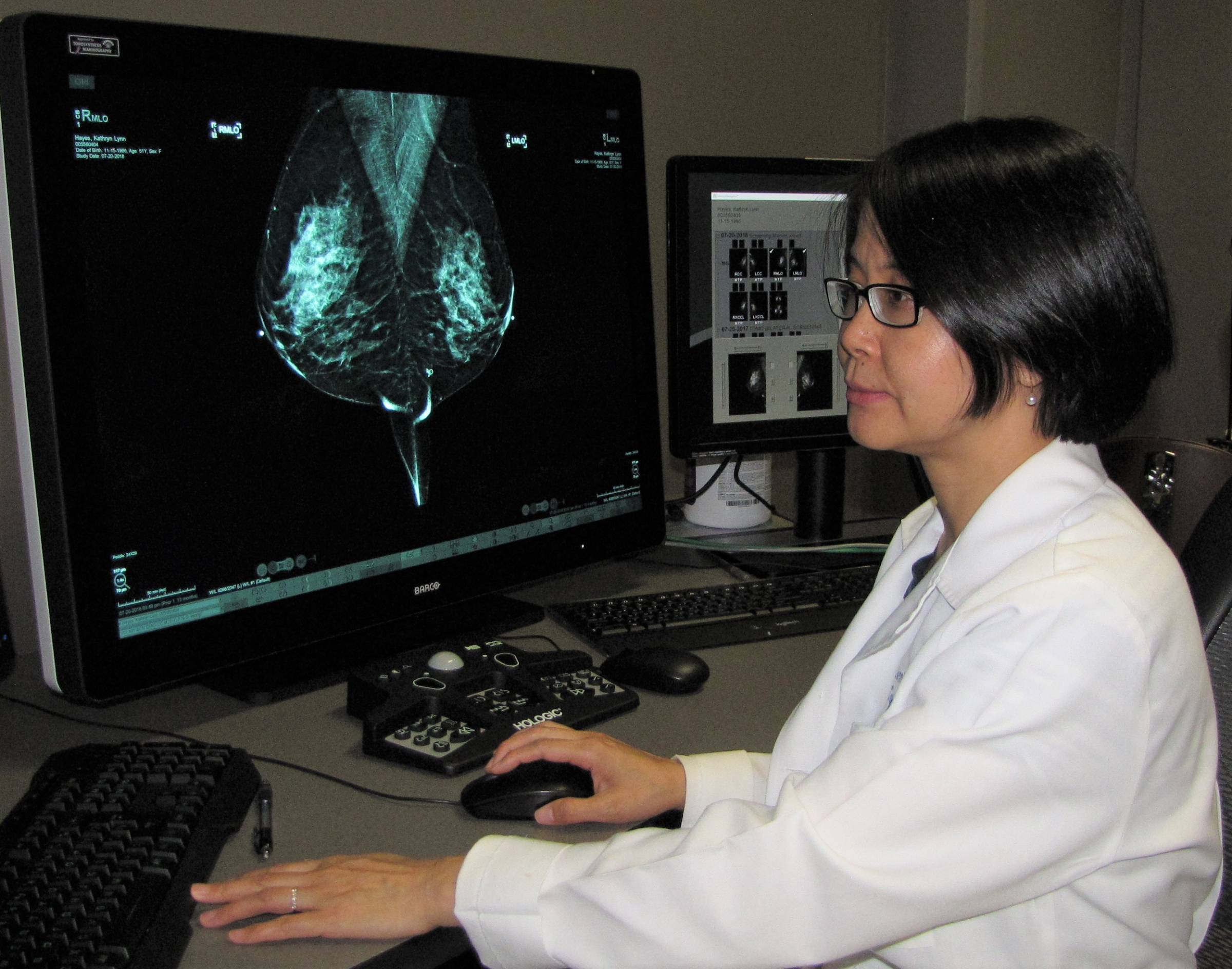
Automatic Annotation of Medical Images for AI Model Training, Xiaoqin Wang, MD
Artificial Intelligence (AI), especially deep learning, has demonstrated revolutionary ability in various medical imaging analysis tasks. However, AI application in medical imaging is limited by the lack of annotated image data. Imaging annotation by experts is expensive and a new tool is needed for automatic image annotation. In this work, we propose a novel, weakly-supervised, self training computer network for breast cancer annotation on mammograms. Our results show our model has improved performance compared to other approaches trained similarly. This new tool can potentially reduce the annotation burden on human experts and can accelerate AI research in medical imaging.

Examples for AI detection of breast cancer on mammograms. Red boxes are bounding boxes for tumor location provided automatically by NLP interpretation of Radiological report and unavailable to AI algorithm. The heatmaps show the AI-predicted location of tumor corresponding closely to the radiologist's label.